The Pitfall of More Powerful Autoencoders in Lidar-Based Navigation
Authors:
C. Gebauer, M. BennewitzType:
PreprintPublished in:
arxiv PreprintYear:
2021Links:
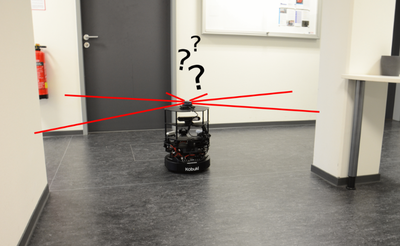
Abstract:
The benefit of pretrained autoencoders for reinforcement learning in comparison to training on raw observations is already known [1]. In this paper, we address the generation of a compact and information-rich state representation. In particular, we train a variational autoencoder for 2D-lidar scans to use its latent state for reinforcement learning of navigation tasks. To achieve high reconstruction power of our autoencoding pipeline, we propose an - in the context of autoencoding 2D-lidar scans - novel preprocessing into a local binary occupancy image. This has no additional requirements, neither self-localization nor robust mapping, and therefore can be applied in any setting and easily transferred from simulation in real-world. In a second stage, we show the usage of the compact state representation generated by our autoencoding pipeline in a simplistic navigation task and expose the pitfall that increased reconstruction power will always lead to an improved performance. We implemented our approach in python using tensorflow. Our datasets are simulated with pybullet as well as recorded using a slamtec rplidar A3. The experiments show the significantly improved reconstruction capabilities of our approach for 2D-lidar scans w.r.t. the state of the art. However, as we demonstrate in the experiments the impact on reinforcement learning in lidar-based navigation tasks is non-predictable when improving the latent state representation generated by an autoencoding pipeline. This is surprising and needs to be taken into account during the process of optimizing a pretrained autoencoder for reinforcement learning tasks.