Deep Reinforcement Learning for Next-Best-View Planning in Agricultural Applications
Authors:
X. Zeng, T. Zaenker, M. BennewitzType:
Conference ProceedingPublished in:
IEEE International Conference on Robotics & Automation (ICRA)Year:
2022Related Projects:
Phenorob - Robotics and Phenotyping for Sustainable Crop ProductionLinks:
BibTex String
@inproceedings{zeng22icra,
title={Deep reinforcement learning for next-best-view planning in agricultural applications},
author={Zeng, Xiangyu and Zaenker, Tobias and Bennewitz, Maren},
booktitle={Proc. of. the International Conference on Robotics and Automation (ICRA)},
year={2022}
}
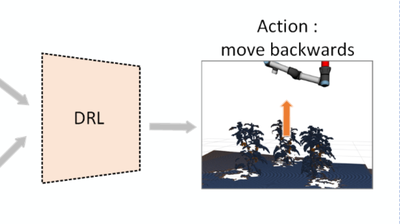
Abstract:
Automated agricultural applications, i.e., fruit picking require spatial information about crops and, especially, their fruits. In this paper, we present a novel deep reinforcement learning (DRL) approach to determine the next best view for automatic exploration of 3D environments with a robotic arm equipped with an RGB-D camera. We process the obtained images into an octree with labeled regions of interest (ROIs), i.e., fruits. We use this octree to generate 3D observation maps that serve as encoded input to the DRL network. We hereby do not only rely on known information about the environment, but explicitly also represent information about the unknown space to force exploration. Our network takes as input the encoded 3D observation map and the temporal sequence of camera view pose changes, and outputs the most promising camera movement direction. Our experimental results show an improved ROI targeted exploration performance resulting from our learned network in comparison to a state-of-the-art method.