Fruit Mapping with Shape Completion for Autonomous Crop Monitoring
Authors:
S. Marangoz, T. Zaenker, R. Menon, M. BennewitzType:
Conference ProceedingPublished in:
IEEE International Conference on Automation Science and Engineering (CASE)Year:
2022Related Projects:
Phenorob - Robotics and Phenotyping for Sustainable Crop ProductionLinks:
BibTex String
@inproceedings{marangoz22case,
title={Fruit mapping with shape completion for autonomous crop monitoring},
author={Marangoz, Salih and Zaenker, Tobias and Menon, Rohit and Bennewitz, Maren},
booktitle={IEEE International Conference on Automation Science and Engineering (CASE)},
year={2022}
}
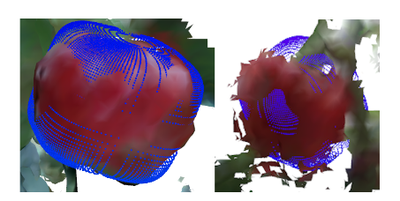
Abstract:
Autonomous crop monitoring is a difficult task due to the complex structure of plants. Occlusions from leaves can make it impossible to obtain complete views about all fruits of, e.g., pepper plants. Therefore, accurately estimating the shape and volume of fruits from partial information is crucial to enable further advanced automation tasks such as yield estimation and automated fruit picking. In this paper, we present an approach for mapping fruits on plants and estimating their shape by matching superellipsoids. Our system segments fruits in images and uses their masks to generate point clouds of the fruits. To combine sequences of acquired point clouds, we utilize a real-time 3D mapping framework and build up a fruit map based on truncated signed distance fields. We cluster fruits from this map and use optimized superellipsoids for matching to obtain accurate shape estimates. In our experiments, we show in various simulated scenarios with a robotic arm equipped with an RGB-D camera that our approach can accurately estimate fruit volumes. Additionally, we provide qualitative results of estimated fruit shapes from data recorded in a commercial glasshouse environment.