Pedestrians and Robots: A Novel Dataset for Learning Distinct Social Navigation Forces
Authors:
S. Agrawal, N. Ostermann-Myrau, N. Dengler, M. BennewitzType:
PreprintPublished in:
Arxiv Pre-printYear:
2025Related Projects:
Embodied AI at LAMARR Institute for Machine Learning and Artificial IntelligenceLinks:
BibTex String
@article{agrawal2025dataset,
title={Pedestrians and Robots: A Novel Dataset for Learning Distinct Social Navigation Forces},
author={Agrawal, Subham and Ostermann-Myrau, Nico and Dengler, Nils and Bennewitz, Maren},
journal={arXiv preprint arXiv:2503.16481},
year={2025}
}
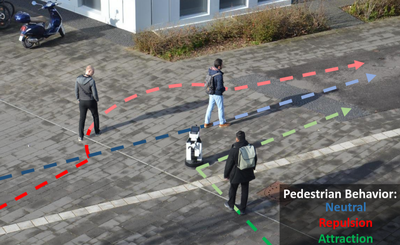
Abstract:
The increasing use of robots in human-centric public spaces such as shopping malls, sidewalks, and hospitals, requires understanding of how pedestrians respond to their presence.However, existing research lacks comprehensive datasets that capture the full range of pedestrian behaviors, e.g., including avoidance, neutrality, and attraction in the presence of robots. Such datasets can be used to effectively learn models capable of accurately predicting diverse responses of pedestrians to robot presence, which are crucial for advancing robot navigation strategies and optimizing pedestrian-aware motion planning.In this paper, we address these challenges by collecting a novel dataset of pedestrian motion in two outdoor locations under three distinct conditions, i.e., no robot presence, a stationary robot, and a moving robot.Thus, unlike existing datasets, ours explicitly encapsulates variations in pedestrian behavior across the different robot conditions.Using our dataset, we propose a novel Neural Social Robot Force Model~(NSRFM), an extension of the traditional Social Force Model that integrates neural networks and robot-induced forces to better predict pedestrian behavior in the presence of robots.We validate the NSRFM by comparing its generated trajectories on different real-world datasets. Furthermore, we implemented it in simulation to enable the learning and benchmarking of robot navigation strategies based on their impact on pedestrian movement. Our results demonstrate the model’s effectiveness in replicating real-world pedestrian reactions and its its utility in developing, evaluating, and benchmarking social robot navigation algorithms.