Context-Based Meta Reinforcement Learning for Robust and Adaptable Peg-in-Hole Assembly Tasks
Authors:
A. Shokry, W. Gomaa, T. Zaenker, M. Dawood, R. Menon, S. Maged, M. Awad, M. BennewitzType:
PreprintPublished in:
Arxiv Pre-printYear:
2025Funding:
LAMARR Institute for Machine Learning and Artificial IntelligenceRelated Projects:
Embodied AI at LAMARR Institute for Machine Learning and Artificial Intelligence, Robotics Institute GermanyLinks:
BibTex String
@article{shokry2025contextbasedmetarL,
title={Context-Based Meta Reinforcement Learning for Robust and Adaptable Peg-in-Hole Assembly Tasks},
author={Ahmed Shokry and Walid Gomaa and Tobias Zaenker and Murad Dawood and Shady A. Maged and Mohammed I. Awad and Maren Bennewitz},
journal={arXiv preprint arXiv:2409.16208},
year={2025}
}
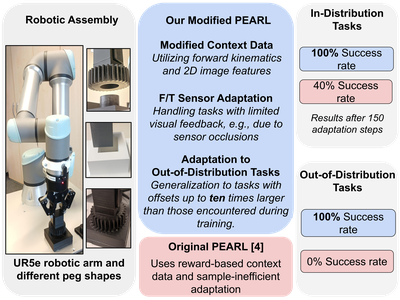
Abstract:
Autonomous assembly is an essential capability for industrial and service robots, with Peg-in-Hole (PiH) insertion being one of the core tasks. However, PiH assembly in unknown environments is still challenging due to uncertainty in task parameters, such as the hole position and orientation, resulting from sensor noise. Although context-based meta reinforcement learning (RL) methods have been previously presented to adapt to unknown task parameters in PiH assembly tasks, the performance depends on a sample-inefficient procedure or human demonstrations. Thus, to enhance the applicability of meta RL in real-world PiH assembly tasks, we propose to train the agent to use information from the robot's forward kinematics and an uncalibrated camera. Furthermore, we improve the performance by efficiently adapting the meta-trained agent to use data from force/torque sensor. Finally, we propose an adaptation procedure for out-of-distribution tasks whose parameters are different from the training tasks. Experiments on simulated and real robots prove that our modifications enhance the sample efficiency during meta training, real-world adaptation performance, and generalization of the context-based meta RL agent in PiH assembly tasks compared to previous approaches.