Centroidal State Estimation based on the Koopman Embedding for Dynamic Legged Locomotion
Authors:
S. Khorshidi, M. Dawood, M. BennewitzType:
Conference ProceedingPublished in:
IEEE/RSJ International Conference on Intelligent Robots and Systems (IROS)Year:
2024Related Projects:
Embodied AI at LAMARR Institute for Machine Learning and Artificial Intelligence, Phenorob - Robotics and Phenotyping for Sustainable Crop ProductionDOI:
https://doi.org/10.1109/IROS58592.2024.10801750Links:
BibTex String
@inproceedings{khorshidi24iros,
title={Centroidal State Estimation based on the Koopman Embedding for Dynamic Legged Locomotion},
author={Khorshidi, Shahram and Dawood, Murad and Bennewitz, Maren},
booktitle={Proc. of the IEEE/RSJ Int. Conf. on Intelligent Robots and Systems (IROS)},
year={2024}
}
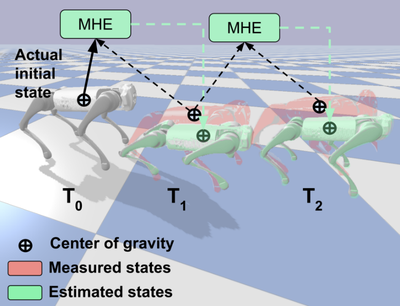
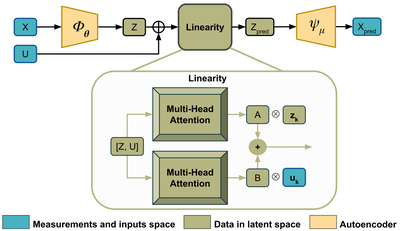
Abstract:
In this paper, we introduce a novel approach to centroidal state estimation, which plays a crucial role in predictive model-based control strategies for dynamic legged locomotion. Our approach uses the Koopman operator theory to transform the robot’s complex nonlinear dynamics into a linear system, by employing dynamic mode decomposition and deep learning for model construction. We evaluate both models on their linearization accuracy and capability to capture both fast and slow dynamic system responses. We then select the most suitable model for estimation purposes, and integrate it within a moving horizon estimator. This estimator is formulated as a convex quadratic program, to facilitate robust, real-time centroidal state estimation. Through extensive simulation experiments on a quadruped robot executing various dynamic gaits, our data-driven framework outperforms conventional filtering techniques based on nonlinear dynamics. Our estimator addresses challenges posed by force/torque measurement noise in highly dynamic motions and accurately recovers the centroidal states, demonstrating the adaptability and effectiveness of the Koopman-based linear representation for complex locomotive behaviors. Importantly, our model based on dynamic mode decomposition, trained with two locomotion patterns (trot and jump), successfully estimates the centroidal states for a different motion (bound) without retraining.