Map Space Belief Prediction for Manipulation-Enhanced Mapping
Authors:
J.M.C Marques, N. Dengler, T. Zaenker, J. Mücke, S. Wang, M. Bennewitz, K. HauserType:
PreprintPublished in:
Accepted to Robotics: Science and Systems (RSS)Year:
2025Links:
BibTex String
@inproceedings{marques25rss,
title={Map Space Belief Prediction for Manipulation-Enhanced Mapping},
author={ Marques, Joao Marcos Correia and Dengler, Nils and Zaenker, Tobias and Muecke, Jesper and Shenlong, Wang and Bennewitz, Maren and Hauser, Kris},
journal={Proc.~of Robotics: Science and Systems (RSS)},
year={2025} }
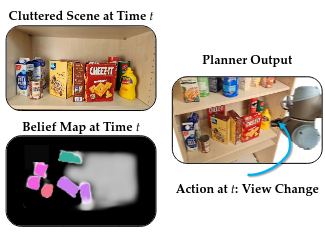
Abstract:
Searching for objects in cluttered environments requires selecting efficient viewpoints and manipulation actions to remove occlusions and reduce uncertainty in object locations, shapes, and categories.In this work, we address the problem of manipulation-enhanced semantic mapping, where a robot has to efficiently identify all objects in a cluttered shelf.Although Partially Observable Markov Decision Processes~(POMDPs) are standard for decision-making under uncertainty, representing unstructured interactive worlds remains challenging in this formalism.To tackle this, we define a POMDP whose belief is summarized by a metric-semantic grid map and propose a novel framework that uses neural networks to perform map-space belief updates to reason efficiently and simultaneously about object geometries, locations, categories, occlusions, and manipulation physics. Further, to enable accurate information gain analysis, the learned belief updates should maintain calibrated estimates of uncertainty. Therefore, we propose Calibrated Neural-Accelerated Belief Updates (CNABUs) to learn a belief propagation model that generalizes to novel scenarios and provides confidence-calibrated predictions for unknown areas. Our experiments show that our novel POMDP planner improves map completeness and accuracy over existing methods in challenging simulations and successfully transfers to real-world cluttered shelves in zero-shot fashion.